
Compared with the radiologists, the method proposed by this paper has a better performance. Some study shows that the accuracy of differentiating GGNs’ invasiveness by experienced radiologists is about 80%. So, the three-dimensional convolutional neural network (3D-CNN) is used to undertake this task. PILs have nearly 100% disease-specific survival, and the IAs’ disease-specific survival is greatly reduced.ĭeep learning has been proven to have great advantages over traditional machine learning methods in image classification and target detection. The AAH, AIS, and MIA are regarded as preinvasive lesion (PIL). In 2011, the International Association for the Study of Lung Cancer (IASLC), American Thoracic Society (ATS), and European Respiratory Society (ERS) classified small (< 3 cm), solitary adenocarcinomas into four main types: atypical adenomatous hyperplasias (AAH), adenocarcinoma in situ (AIS), minimally invasive adenocarcinoma (MIA), and invasive adenocarcinomas (IA). The invasiveness categories of lung adenocarcinoma appearing as GGN have become more and more detailed in the latest classification standards. Therefore, this paper focuses on the accurate identification of this GGNs’ invasiveness. Whether the patient is diagnosed with preinvasive lesion (PIL) or invasive adenocarcinoma (IA) has a significant effect on the prognosis and disease-specific survival. The accuracy of pathological classification is always crucial for the further treatment. There are similar HRCT manifestations at GGNs’ different development stages. The adenocarcinoma appearing as GGN has a development from non-solid to solid.
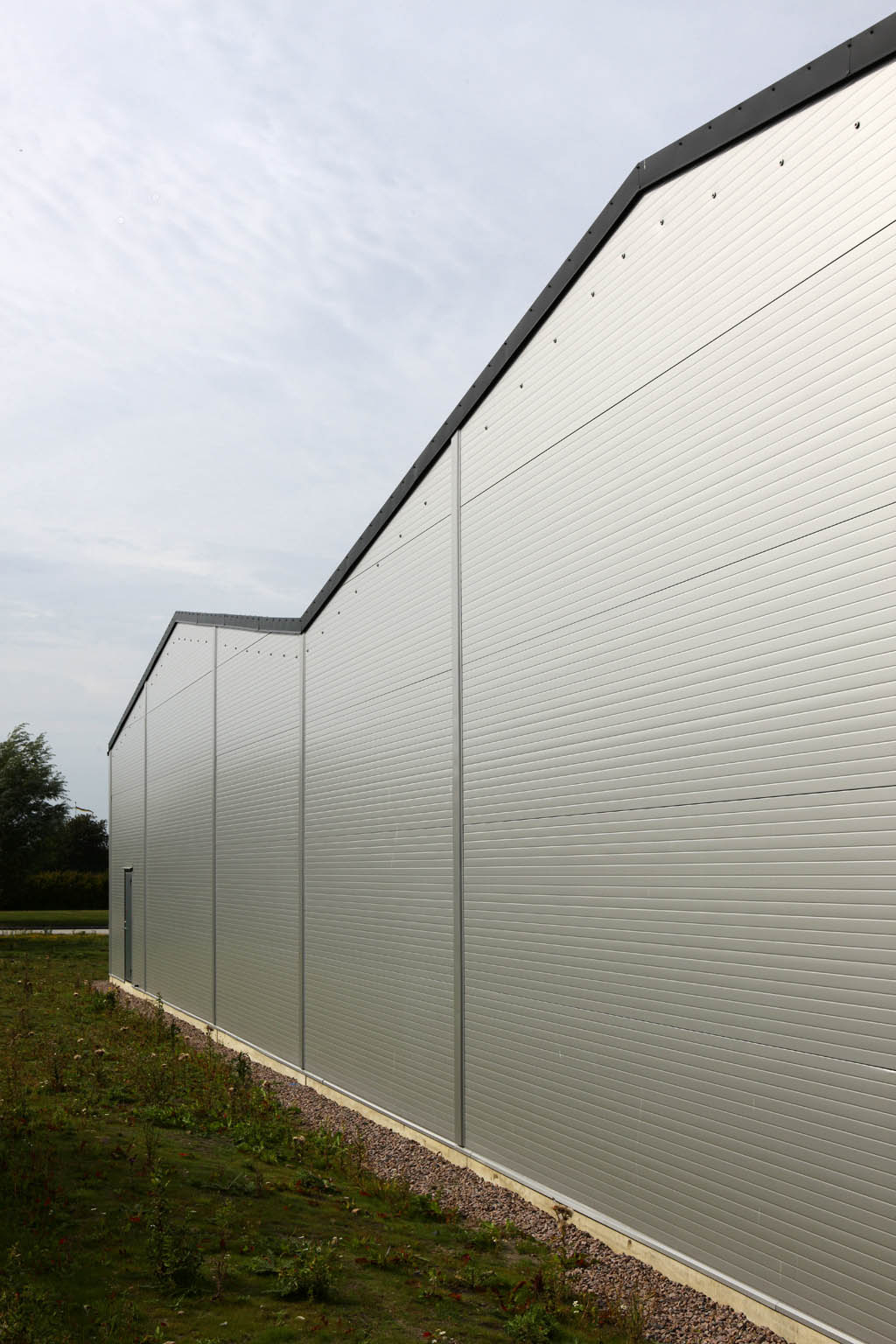

Although GGNs are slow-growing lesions, recent studies have shown that GGNs are closely related to the early stage of lung cancer. With the increasing popularization of low-dose CT (LDCT), numerous pulmonary nodules appearing as ground-glass nodules (GGNs) are detected. The automatic GGN detector with the addition of Attention-v1 can be used to construct the GGN invasiveness classification algorithm to help the patients and doctors in treatment. Attention mechanism improves 3D-CNN’s performance compared with the residual block. The deep 3D-CNN’s classification result is better than traditional machine learning method. The algorithm proposed in this paper outperforms ResNet and random forest in sensitivity, accuracy, and AUC value. The accuracy, sensitivity, specificity, and area under curve (AUC) value of Attention-v1 structure are 85.2%, 83.7%, 86.3%, and 92.6%. The competition performance metrics (CPM) of automatic GGN detector reached 0.896. ResNet is one of the most advanced convolutional neural network structures.
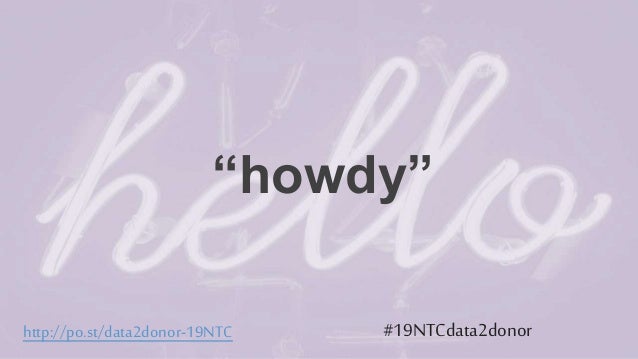
This paper conducted a contract experiment to compare the performance of Attention-v1, ResNet, and random forest algorithm. The attention mechanism was introduced to the 3D-CNN.

Then, a deep 3D convolutional neural network (3D-CNN) called Attention-v1 is used to identify the GGNs’ invasiveness. Firstly, the automatic GGN detector which is composed by a 3D U-Net and a 3D multi-receptive field (multi-RF) network detects the location of GGNs. The data is in high-resolution computed tomography (HRCT) format. 1431 clinical cases and a total of 1624 GGNs (3–30 mm) were collected from Shanghai Cancer Center for the study. This paper proposes an automatic GGNs’ invasiveness classification algorithm for the adenocarcinoma. The diagnosis of GGN as preinvasive lesion (PIL) or invasive adenocarcinoma (IA) is very important for further treatment planning. The early stage lung cancer often appears as ground-glass nodules (GGNs).
